Are you tired of scratching your head in confusion every time someone mentions p-values? Well, fret no more, because in this article, we have got you covered.
We will walk you through the definition, formula, table, finding p-value, and significance of p-values. Understanding p-values is crucial for any researcher or data analyst, as they play a key role in determining the strength of evidence against the null hypothesis.
So, if you want to unlock the secrets of p-values and gain the confidence to interpret your test results with ease, keep reading to uncover the hidden treasures of statistical significance.
P-value Calculation Methods
There are several methods available for calculating p-values, which determine the statistical significance of test results. One common approach is manual calculation, where the p-value is derived from the z-score, t-score, or chi-square value obtained from various tests.
Another option is to use statistical software or spreadsheets like Excel to calculate p-values based on the score and level of significance.
To find the p-value, you can refer to tables specifically designed for t-scores, z-scores, or chi-square values. These tables allow you to match your test score with the corresponding p-value. If your test statistic is positive, you determine the probability less than the test score and double it to find the p-value. Conversely, if the test statistic is negative, you determine the probability greater than the test score and also double it to find the p-value.
P-values play a crucial role in interpreting test results. They provide a universal language for comparing results from different tests and help determine if there’s enough evidence to reject the null hypothesis. Ranging from 0 to 1, p-values can be interpreted as the statistical significance of the results.
In specific tests like t-tests, z-tests, or chi-square tests, you can find the p-value by using the corresponding table and the test score. Each test has its own formula for calculating the p-value, which depends on the type of statistical test being conducted.
P-value Tables
Now let’s turn our attention to the topic of P-value tables.
P-value tables play a crucial role in interpreting test results and determining the significance of findings.
These tables allow you to match your test scores with the corresponding p-values, providing a standardized way to measure the strength of evidence against the null hypothesis.
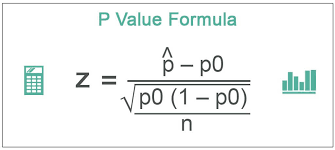
Table Interpretation
P-value tables provide a convenient tool for determining the statistical significance of test results by matching test scores with corresponding p-values. These tables contain a range of test scores and their corresponding p-values for different levels of significance.
To interpret the table, locate the test score obtained from your statistical test and find the corresponding p-value. The p-value represents the probability of obtaining a test score as extreme as the observed data, assuming the null hypothesis is true. The smaller the p-value, the stronger the evidence against the null hypothesis.
Generally, if the p-value is less than the chosen level of significance (commonly 0.05), the results are considered statistically significant. P-value tables allow researchers to determine the significance of their findings and make informed decisions based on the evidence provided by their data.
P-value Lookup
To utilize P-value tables effectively, you can refer to these tables to determine the statistical significance of your test results. P-value tables provide the critical values for different levels of significance and degrees of freedom.
By comparing your test statistic (such as z-score or t-score) with the corresponding critical value in the table, you can find the p-value. If your test statistic falls within the critical region (i.e., beyond the critical value), the p-value will be smaller, indicating stronger evidence against the null hypothesis.
On the other hand, if your test statistic falls within the non-critical region, the p-value will be larger, suggesting weaker evidence against the null hypothesis.
P-value tables are valuable tools for researchers in determining the significance of their findings and making informed decisions based on the results of their statistical tests.
Significance Determination
Understanding the significance of your results can be determined using P-value tables. These tables provide a convenient way to interpret the statistical significance of your findings. By comparing your test statistic, such as the z-score or t-score, with the corresponding values in the P-value table, you can determine the likelihood of observing the results under the null hypothesis.
The P-value represents the probability of obtaining results as extreme as the observed data, assuming the null hypothesis is true. A small P-value indicates strong evidence against the null hypothesis, while a larger P-value suggests weak evidence.
P-value tables are available for different statistical tests, such as t-tests, z-tests, and chi-square tests, allowing you to determine the significance of your results accurately and efficiently.
Manual Calculation Vs. Statistical Software
When it comes to calculating p-values, you have two options: manual calculation or using statistical software.
Each method has its pros and cons.
Manual calculation allows for a deeper understanding of the underlying statistical concepts and can be useful in educational settings, but it can be time-consuming and prone to human error.
On the other hand, statistical software offers convenience, speed, and accuracy, but it may require a learning curve and reliance on external tools.
Ultimately, the choice between manual calculation and statistical software depends on your specific needs and preferences.
Calculation Methods: Manual Vs Software
One can choose between manual calculation and statistical software when calculating p-values for statistical tests.
Manual calculation involves using p-value tables or spreadsheets like Excel to determine the p-value based on the test score and level of significance. P-value tables exist for t-score, z-score, and chi-square, allowing for easy determination of the corresponding p-value. When using these tables, the test score is matched to the table to find the probability associated with it. If the test statistic is positive, the probability less than the test score is determined and doubled to find the p-value. If the test statistic is negative, the probability greater than the test score is determined and doubled.
On the other hand, statistical software automates the calculation process, making it faster and more accurate. It can handle complex calculations and provides additional features like graphical representation of results. Statistical software can be particularly useful when dealing with large datasets or multiple statistical tests.
Pros and Cons
To evaluate the advantages and disadvantages of manual calculation versus statistical software for determining p-values, it’s important to consider various factors.
Manual calculation allows for a deeper understanding of the underlying statistical concepts and calculations involved. It also provides greater control and flexibility in adjusting calculations based on specific requirements. However, manual calculation can be time-consuming and prone to human error, especially when dealing with complex statistical tests and large datasets.
On the other hand, statistical software offers convenience, speed, and accuracy in calculating p-values. It automates the calculation process and provides built-in functions and algorithms for various statistical tests. However, reliance on software may limit understanding of the underlying calculations and assumptions, and may also lead to blind acceptance of results without critical evaluation.
Ultimately, the choice between manual calculation and statistical software depends on the specific needs, resources, and expertise of the researcher or analyst.
Finding P-value Using Tables
P-values can be conveniently determined by referring to tables corresponding to the specific statistical test being conducted. These tables, such as the t-score table, z-score table, and chi-square table, provide a quick and easy way to find the p-value associated with a particular test statistic. To use these tables, you need to locate the row that corresponds to the degrees of freedom or sample size, and then find the column that matches the test statistic. The intersection of these row and column values will give you the p-value.
When using these tables, it’s important to consider the directionality of the test statistic. If the test statistic is positive, you’ll need to find the probability less than the test score and then double it to obtain the p-value. On the other hand, if the test statistic is negative, you’ll need to find the probability greater than the test score and again double it to find the p-value.
P-value Significance and Interpretation
When interpreting statistical test results, the significance of the p-value provides valuable insight into the strength of evidence against the null hypothesis. The p-value represents the probability of obtaining results as extreme as the observed data, assuming the null hypothesis is true.
It ranges from 0 to 1, with smaller values indicating stronger evidence against the null hypothesis. A p-value less than the chosen significance level (often 0.05) suggests that the observed results are unlikely to occur by chance alone, leading to the rejection of the null hypothesis.
On the other hand, a p-value greater than the significance level indicates that the observed results could plausibly occur by chance, leading to the acceptance of the null hypothesis. It’s important to note that statistical significance doesn’t imply practical significance or the importance of the observed effect. Therefore, it’s crucial to consider the context and practical implications when interpreting the results.
Additionally, p-values allow for easier comparison of results from different tests, enabling researchers to assess the strength of evidence against the null hypothesis across various studies.
Comparing Results With P-Values
Comparing the results of different tests becomes easier and more informative with the use of p-values. P-values provide a universal language for interpreting test results, allowing for a standardized way to evaluate the statistical significance of the findings. By comparing the p-values obtained from different tests, you can determine which results are more statistically significant.
When comparing p-values, it’s important to consider the level of significance chosen for each test. A smaller p-value indicates stronger evidence against the null hypothesis and greater statistical significance. Therefore, if one test yields a p-value of 0.01 and another test yields a p-value of 0.05, the result with the p-value of 0.01 is considered more significant.
Additionally, comparing p-values can help identify patterns or trends across different tests. If multiple tests produce p-values that are consistently small, it provides stronger evidence against the null hypothesis and increases confidence in the findings.
Testing Hypotheses With P-Values
To further explore the significance of test results, it’s important to understand how hypotheses can be tested using p-values.
When conducting hypothesis testing, the p-value is a crucial metric that determines the strength of evidence against the null hypothesis. It represents the probability of obtaining results as extreme as the observed data, assuming the null hypothesis is true.
To test a hypothesis using p-values, you need to calculate the test statistic (such as z-score, t-score, or chi-square value) and compare it to the corresponding p-value table. If the test statistic is positive, you determine the probability less than the test score and double it to find the p-value. Conversely, if the test statistic is negative, you determine the probability greater than the test score and double it to find the p-value.
Once you have obtained the p-value, you can assess the statistical significance of your results. P-values range from 0 to 1: smaller values indicate stronger evidence against the null hypothesis.
To determine whether the null hypothesis should be rejected, you compare the p-value to a predetermined level of significance (often denoted as α). If the p-value is less than α, you can reject the null hypothesis in favor of the alternative hypothesis. On the other hand, if the p-value is greater than α, you fail to reject the null hypothesis.
P-value Range and Interpretation
The range of p-values is between 0 and 1, and they’re interpreted as the statistical significance of the results. A p-value close to 0 indicates strong evidence against the null hypothesis, suggesting that the observed data is unlikely to occur by chance alone. On the other hand, a p-value close to 1 suggests weak evidence against the null hypothesis, indicating that the observed data is likely to occur by chance.
It’s important to note that a p-value doesn’t provide information about the effect size or the practical significance of the results. Instead, it focuses solely on the statistical significance. Generally, a p-value less than 0.05 (or 0.01) is considered statistically significant, indicating that the results are unlikely to be due to random chance. However, the interpretation of p-values should also take into account the specific context and the field of study.
Therefore, it’s crucial to consider the p-value alongside other factors such as effect size, sample size, and the overall research question.
P-Value From T-Test
After understanding the range and interpretation of p-values, the next step is to explore how to calculate the p-value from a t-test.
The p-value from a t-test can be found by using the t-score and the corresponding table. The t-score is calculated by taking the difference between the sample mean and the hypothesized population mean, and dividing it by the standard error of the sample mean.
Once the t-score is obtained, it can be matched with the degrees of freedom in the t-table to determine the corresponding p-value.
The p-value represents the probability of obtaining results as extreme as the observed data, assuming the null hypothesis is true. If the p-value is less than the chosen level of significance (often 0.05), it suggests there’s strong evidence against the null hypothesis and the results are statistically significant.
On the other hand, if the p-value is greater than the chosen level of significance, it suggests there isn’t enough evidence to reject the null hypothesis.
Understanding how to calculate the p-value from a t-test is crucial in determining the statistical significance of the results and making informed decisions based on the analysis.
P-Value From Z-Test
Now let’s delve into the topic of P-Value From Z-Test.
In this subtopic, we’ll discuss the calculation and interpretation of p-values obtained from the z-test.
We’ll also explore how p-values can be compared with the significance level to determine the statistical significance of the results.
Calculation and Interpretation
To calculate and interpret the p-value from a z-test, use the corresponding z-score and table. The p-value represents the probability of obtaining results as extreme as the observed data, assuming the null hypothesis is true. It indicates the strength of evidence against the null hypothesis.
To calculate the p-value, first determine the test statistic (z-score). Then, find the corresponding value in the z-table. If the test statistic is positive, double the probability less than the test score to find the p-value. If the test statistic is negative, double the probability greater than the test score.
The p-value ranges from 0 to 1, with smaller values indicating stronger evidence against the null hypothesis.
Comparison With Significance Level
When comparing the p-value obtained from a z-test to the significance level, it can provide insight into the statistical significance of the results.
The significance level, also known as alpha, is predetermined by the researcher and represents the threshold for rejecting the null hypothesis.
If the p-value is smaller than the significance level, typically set at 0.05, it suggests that the observed data is statistically significant and provides evidence against the null hypothesis.
In this case, you’d reject the null hypothesis and conclude that there’s a significant effect or relationship.
On the other hand, if the p-value is larger than the significance level, it indicates that the observed data isn’t statistically significant, and you’d fail to reject the null hypothesis.
Therefore, comparing the p-value to the significance level allows you to determine the statistical significance of your results.
P-Value From Chi-Square Test
The p-value from a chi-square test is a statistical measure that determines the strength of evidence against the null hypothesis in a categorical data analysis. It helps you determine if there’s enough evidence to reject the null hypothesis and accept the alternative hypothesis.
To calculate the p-value from a chi-square test, you need to compare the chi-square value obtained from the test with the chi-square distribution table. This table provides critical values for different levels of significance and degrees of freedom.
Once you have the chi-square value, you can find the corresponding p-value from the chi-square distribution table. The p-value represents the probability of obtaining results as extreme as the observed data, assuming the null hypothesis is true. Smaller p-values indicate stronger evidence against the null hypothesis.
If the calculated p-value is smaller than the chosen level of significance (usually 0.05), then you can reject the null hypothesis and conclude that there’s a significant relationship between the variables being analyzed.
On the other hand, if the p-value is larger than the chosen level of significance, you fail to reject the null hypothesis and conclude that there isn’t enough evidence to support a significant relationship between the variables.
Definition and Formula of P-value
The formula for calculating the p-value varies depending on the specific statistical test being conducted. Different tests have their own formulas for calculating the p-value.
In general, the p-value represents the probability of obtaining results as extreme as the observed data, assuming the null hypothesis is true. It ranges from 0 to 1, with smaller values indicating stronger evidence against the null hypothesis.
For example, in a t-test, the p-value can be found using the t-score and the corresponding table. Similarly, in a z-test, the p-value can be determined using the z-score and the corresponding table.
For a chi-square test, the p-value is calculated using the chi-square value and the corresponding table.
These formulas allow researchers to determine the statistical significance of their results and make informed decisions about whether to reject the null hypothesis. By understanding and applying the appropriate formula for calculating the p-value, you can effectively evaluate the strength of evidence against the null hypothesis and interpret the significance of your findings.
Conclusion
In conclusion, understanding and calculating p-values is essential in statistical analysis. They provide a measure of the strength of evidence against the null hypothesis and allow for easy comparison of test results.
By using the appropriate formulas and tables, researchers can determine the p-value for different types of tests. The significance of p-values lies in their ability to determine if there’s enough evidence to reject the null hypothesis.
Overall, p-values are a crucial tool in statistical analysis and interpretation of research findings.
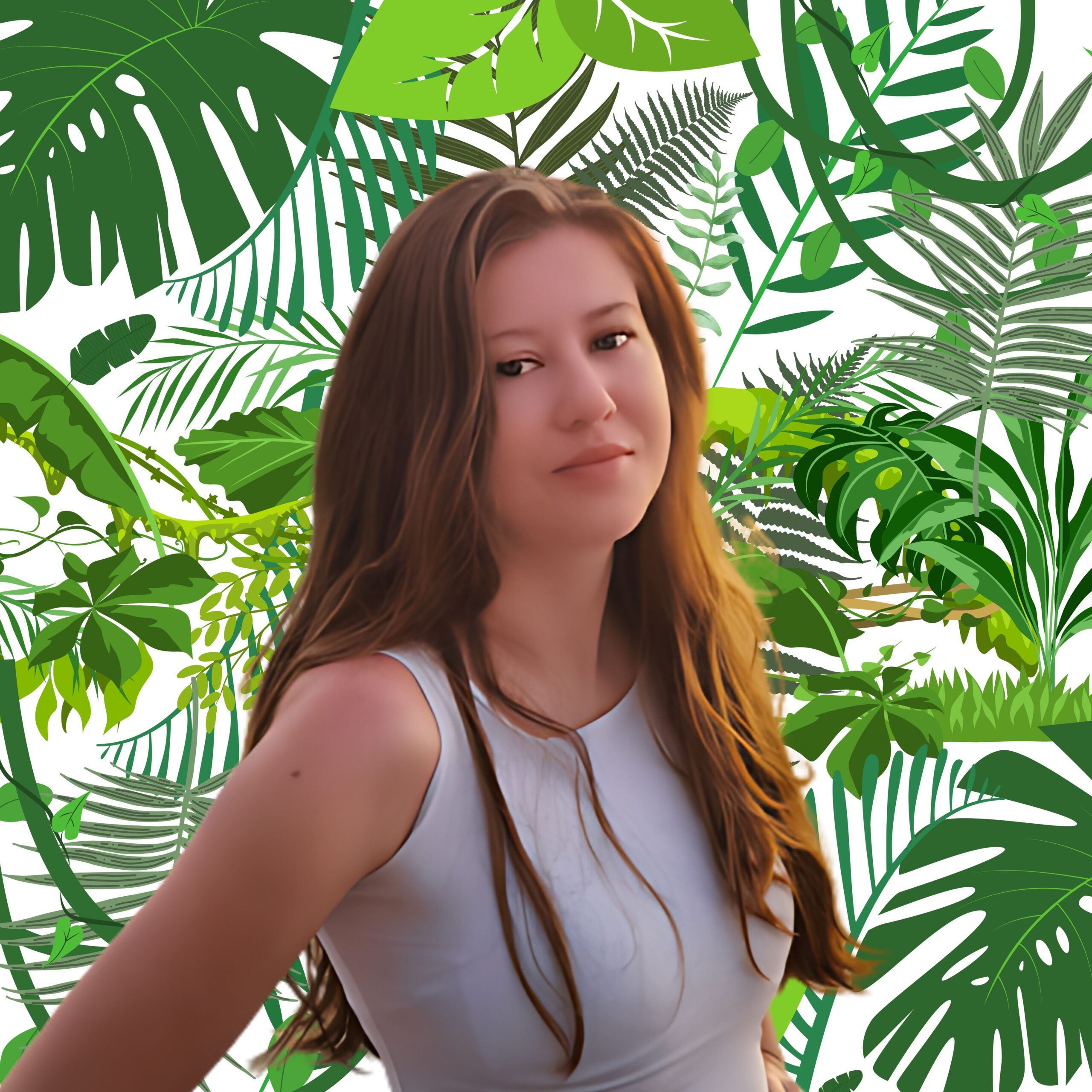
Erzsebet Frey (Eli Frey) is an ecologist and online entrepreneur with a Master of Science in Ecology from the University of Belgrade. Originally from Serbia, she has lived in Sri Lanka since 2017. Eli has worked internationally in countries like Oman, Brazil, Germany, and Sri Lanka. In 2018, she expanded into SEO and blogging, completing courses from UC Davis and Edinburgh. Eli has founded multiple websites focused on biology, ecology, environmental science, sustainable and simple living, and outdoor activities. She enjoys creating nature and simple living videos on YouTube and participates in speleology, diving, and hiking.